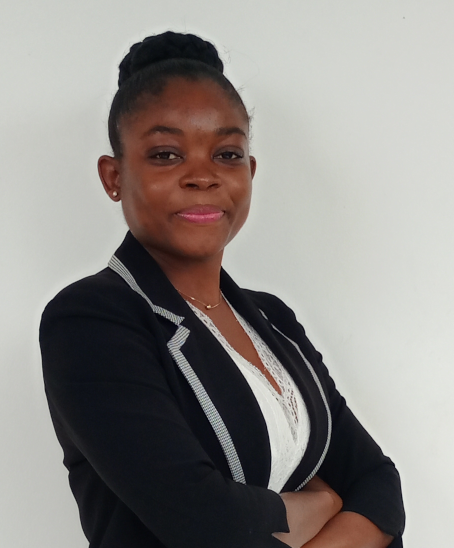
It is often said that the spectacular success of neural networks has been made possible due the abundance of data. In the context of supervised learning and more precisely classification, a quality data over which a model could be trained in earnest has to be balanced, which means the model would need to see relatively the same amount of information on each of the classes during the training process for a better generalisation. While at AIMS, Hortence’s goal was to solve the problem of fraud detection in credit cards by fixing herself on the problem of unbalanced data using machine learning algorithms. To do that, she proposed a novel regularisation strategy that uses two factors which are mean divergence and variance divergence . The proposed terms are added to the loss function, and they are considered as penalties terms to the model against the majority and for the minority class .
Hortence is currently a Master student in Machine Learning and Artificial Intelligence at Stellenbosch University.