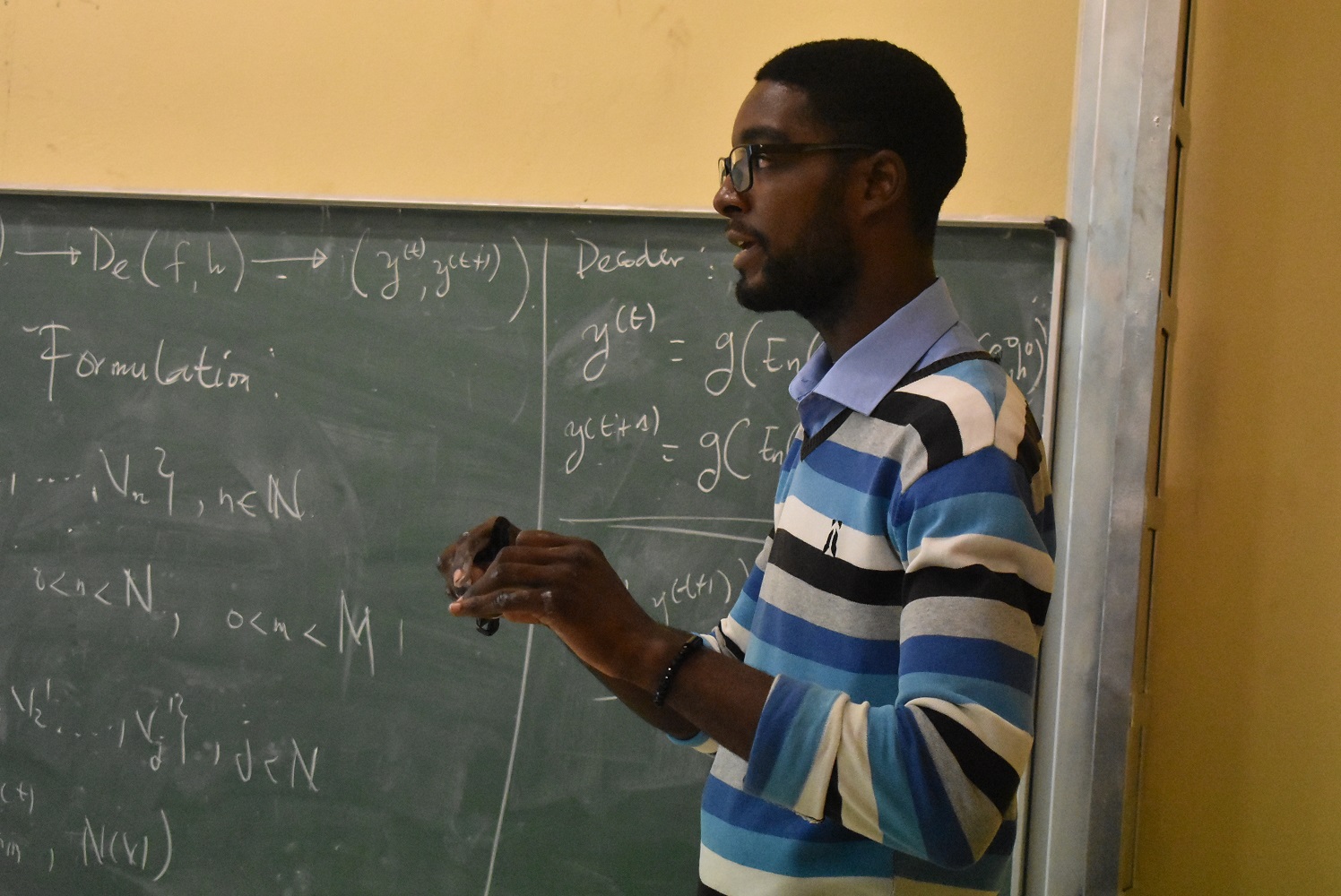
One of the highlights at AIMS Cameroon Centre in Limbe last week was a talk by 8th Cohort Graduate Allassan Nken Tchangmena Tchangmena in the main lecture hall. This fresh graduate from AIMS edified 9th cohort students with a lecture on his research essay titled “Data Prediction in Wireless Sensor Netwotks”
A WSN is a network composed of spatially distributed sensors across a given geographical location called the coverage area. Their main aim is to collect sensing data from the environment (land, water, air, etc). Basically, WSNs are composed of nodes in which the different sensors are attached. The collected information are then channelled either to a central node or to the base station, also known as the Sink.
Allassan Nken said WSNs are commonly used where remote monitoring and control is needed. As such, their application can be found in environmental data collection, smart transportation systems, health monitoring systems, agriculture and so on.
“This is mostly because of their very low cost, abundant availability and large scale deployment capacity. Despite this high usability property, WSN applications come up with many inconveniences like data transmission delay, poor and abnormal transmission quality and very high energy consumption. Energy consumption is indeed one of the commonly encountered problem in WSNs as it has a strong relationship with life-time of the nodes constituting the network. That is to say, the higher the working activity of the network nodes, the faster it deteriorates and as a result the less efficient data transmission becomes, ” explained Allassan.
Related works to overcome this problem rely on techniques such as Data Compression and Data Clustering Algorithms, to limit the quantity of information transmitted.
Recent advancement on research in the field of data science and artificial intelligence has made it possible to develop a new technique called Data Prediction. Fortunately, Data Prediction has proven to be an excellent approach as it improves energy consumption and data transmission efficiency by reconstructing data lost due to node failure.
The main objective of Allasan’s work is to propose a technique of Data Prediction using Machine Learning, that exploits the time distribution property of the data collected by the sensors, to effectively predict unavailable data, within a particular period of time.
Allassan also aims to propose a deep-learning model that exploits the spatio-temporal distribution of the sensing data to accurately reconstruct data loss during nodes failure. Indeed, predicting the sensing data for a set of nodes within a period of time has a crucial advantage that the sensor data collected at the node level will only be transferred if the difference between the predicted value and the observed value of the data is greater than a given threshold state. By doing so, data transmission is limited to a reasonable quantity of information transfer, to the base station.
The contribution of Allassan’s work includes suggesting a model based on a sequence-to-sequence architecture to predict multiple output data of a sensor node, simultaneously; initiating a data pre-processing technique based on Data Denoising and Data Normalization to remove erroneous values and smoothen the data for efficient model training; and evaluating the effectiveness of the model by doing a comparative analysis with another model architecture.